Sijun Tan
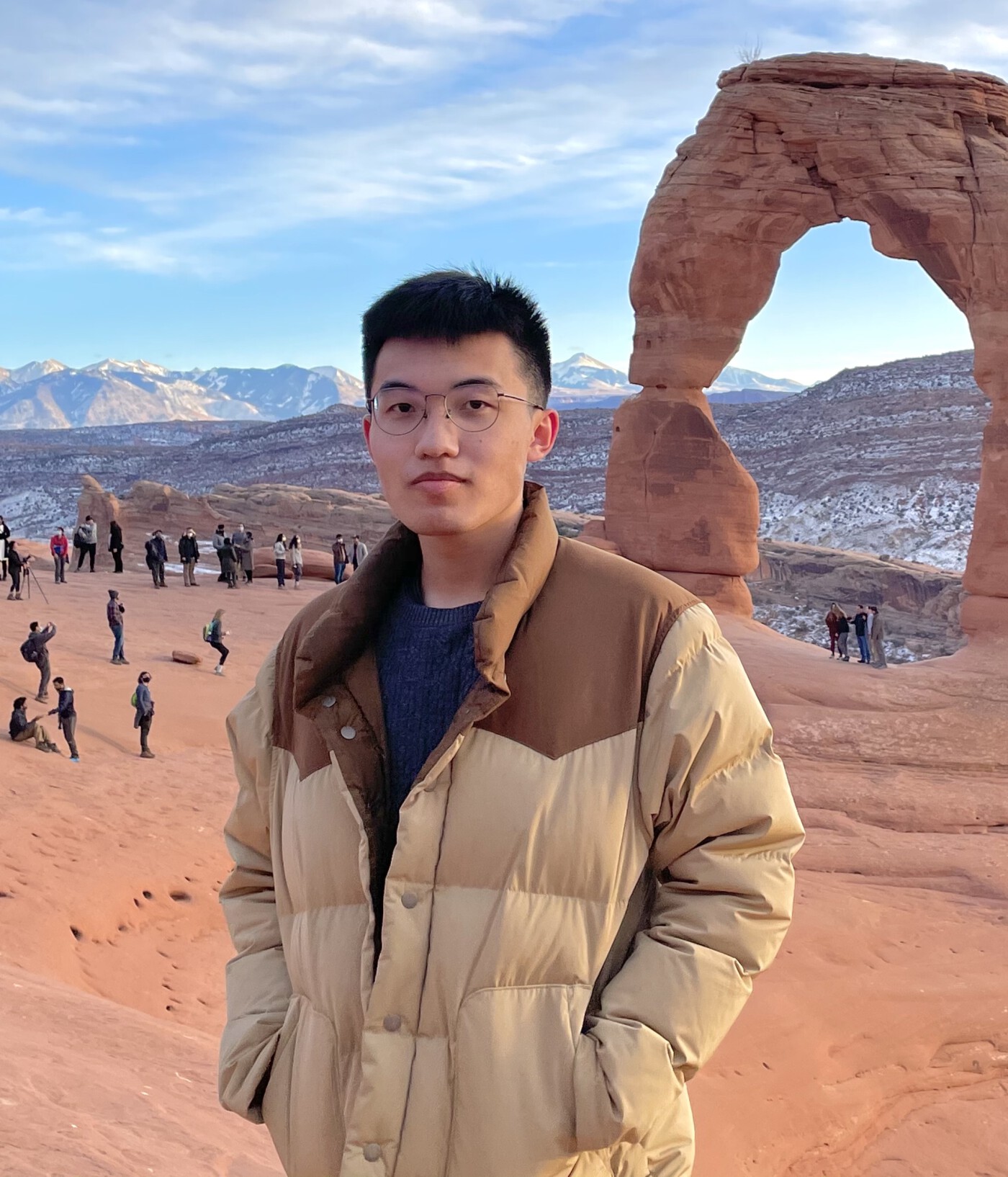
I graduated with highest distinction from the University of Virginia with a Bachelor’s degree in Computer Science and Mathematics. During my undergraduate study, I worked with Professors David Wu and Yuan Tian on scalable privacy-preserving machine learning, and with Professors Haifeng Xu and Xiaohui Bei on handling miscalibration in peer reviews.
My research lies in cryptography, machine learning, and distributed systems. I am mainly interested in research in the following two directions: 1) build systems that can protect users’ data privacy by leveraging cryptographic tools. 2) studying cryptographic primitives that can improve the scalability, verifiability, and privacy of blockchain.
news
Sep 26, 2021 | Our paper Least Square Calibration in Peer Reviews is accepted to appeear at NeurIPS2021. |
---|---|
Jul 14, 2021 | I give a talk on my research work CryptGPU at Stanford Security Lunch. |
Feb 19, 2021 | Our paper CryptGPU: Fast Privacy Preserving Machine Learning on the GPU is accepted to appear at IEEE S&P2021. |
May 19, 2020 | I joined FAIR NY working on building and scaling CrypTen with GPU. |